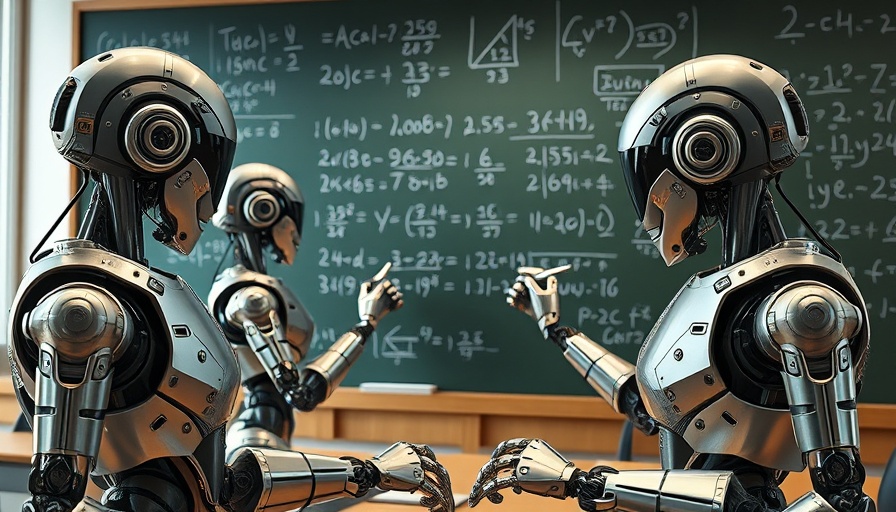
Understanding the Breakthrough: Simple Sampling in LLMs
In a significant development from researchers at Google and UC Berkeley, a new paper highlights a groundbreaking approach to enhancing the reasoning abilities of large language models (LLMs) through a technique called sampling-based search. This research reveals how a deceptively simple test-time mechanism can yield impressive results, particularly for models like Gemini 1.5 Pro, which outperformed established models on various benchmarks without the need for complex training processes.
Why Traditional Models Fall Short
The current landscape of LLM enhancement often leans heavily on intricate architectures and specialized training approaches. Popular methods like reinforcement learning and self-consistency, although effective, typically demand substantial computational resources and training investments. With self-consistency, for instance, the model generates multiple answers and relies on the most frequently appearing one, which can falter under complex queries. This raises a critical question: Are we overcomplicating the development of effective AI systems?
The Simplicity of Sampling-Based Search
By advocating for a minimalist design, the researchers propose that LLMs can generate multiple responses to a query and self-verify them. This innovative method entails the following steps: generating diverse responses using varied prompts, verifying these outputs across multiple iterations, and selecting the most accurate answer based on verification scores. Such a method doesn't only enhance performance but also shows the potential for scalability—allowing models to handle increased workloads simply by generating more responses.
Implications for AI in Franchising
As businesses continue to explore AI integration, particularly within the franchise sector, the insights garnered from this study could usher in a new era of franchise automation. For franchisors and franchisees alike, embracing AI-driven systems can streamline operations, improve customer service, and enhance decision-making processes. Imagine AI managing everything from onboarding to lead generation, utilizing a sampling-based search method to provide tailored solutions efficiently—this could be the catalyst for the next wave of franchise growth.
AI-Driven Business Transformation
This revolutionary approach could also redefine performance benchmarks in franchise technology. By simplifying the training and implementation of AI systems, organizations can invest less time on heavy computational tasks and more on leveraging AI's capabilities for customer insights and predictive analytics. This transition not only fosters innovation but allows for a more agile response to market demands, making adoption more feasible for multi-unit franchises looking to scale rapidly.
The Future of Franchising with AI
As this landscape evolves, franchise leaders must stay ahead of the curve by embracing automated solutions informed by LLM advancements like sampling-based search. The competitive edge lies in foresight and adaptability—essential traits in today's hyperconnected business environment. The paradigm is shifting towards a more integrated, tech-savvy operational model, positioning AI as a critical player in strategic planning and resource allocation.
Conclusion: The Call to Embrace Simplicity
The research from UC Berkeley and Google prompts us to reconsider our assumptions about AI's path to efficacy. As franchises seek to enhance their operations amid increasing competition, exploring simplified AI strategies like sampling-based search could provide the necessary tools for tomorrow's success. Are we ready to rethink our approach to AI integration and unlock the potential it holds for our businesses?
Write A Comment